Writing About Data - Statistical Tests
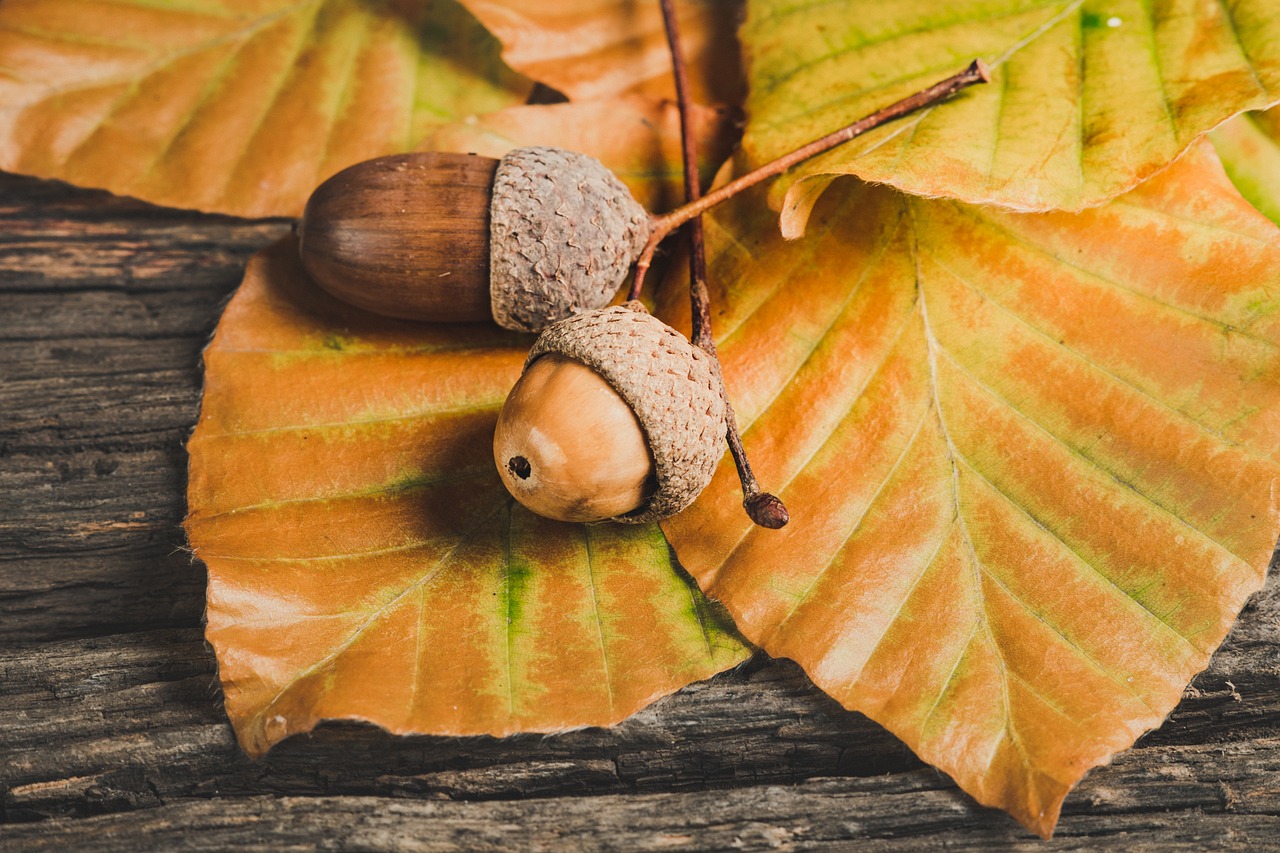
This section provides advice on how to write about the statistical tests you have carried out on your data.
Report all your results
In hypothesis testing, statistical significance is the main criterion for forming conclusions. You compare your calculated p-value to a set critical probability or significance level (usually 0.05) to decide whether your results are statistically significant or non-significant.
You should not present your results as if you ran only the tests presented in the paper if you actually ran more tests. This is because the calculated p-values are interpreted differently if there are a few of them or lots of them.
For example, if you run only two tests on your dataset and report those p-values, it is likely that there really is only a 5% chance that you incorrectly conclude that the null hypothesis is false when in fact it is true (Type I error). However, if you actually ran 50 tests and only reported the two that were statistically significant, it is very likely that you have reported false positives. In other words, the two tests you report may not be statistically significant in other samples of data (of the same size).
So… report ALL the tests you run and don’t discard results you don’t like!
- Most statistical tests make assumptions about your data (such as that your data is normally distributed). You should note that the assumptions were assessed, but the result of each diagnostic test is usually not included.
- Usually, the results of your statistical tests are ordered from most to least important, except when this will disrupt the flow of your story.
- You should report the exact p-values as calculated by the statistical software/website, rather than merely stating that the result is statistically significant or not.
Here are some words that you need to be very careful when using. Many of these words have at least two meanings: a meaning in common talk (eg "normal" means not strange in day-to-day conversation) and a specific statistical meaning (eg "normal" means following a normal distribution).
Use them wisely and properly!
- proved / proven : Proof is only possible within theoretical frameworks such as logic and mathematics. In contrast, scientific empirical research generates evidence. Accumulated evidence from multiple studies may eventually satisfy a “burden of proof,” but individual research projects are rarely definitive so avoid saying you have "proved" something.)
- estimated / derived : We could say that we "estimated" monthly growth rates from a regression formula (we put in a value for "x" and calculated a value for "y"), and we "derived" annual growth rates from the monthly growth estimates (by multiplying by 12). Note the difference.
- risk / probability / likelihood : Probability refers to the chance that a particular outcome occurs based on the values of parameters in a model. Likelihood refers to how well a sample provides support for particular values of a parameter in a model. Risk means the possibility of a potential risk occurring, interpreted using qualitative values such as low, medium, or high.
- significance (statistical or “real world”, parameter or model) : if something has statistical significance, the calculated p-value is less than the significance level (5%). So make sure you use the words "significant" and "significance" carefully.
- normal : This usually refers to the normal distribution, a symmetrical distribution.
- controlling for / adjusting for : The use of “control” leads people to believe that the procedure in question does something stronger than it really does. It is more accurate to “attempt to adjust for” or “attempt to take into account”.
- random (variables, effects) : Don't say "I took measurements in 15 random locations." Do say "I used random sampling techniques to chose 15 independent sites for study." Don't say "My data showed some very random results." Do say "My data had a high level of variance, with a standard deviation of x.x and three outliers."
- False precision. As a general rule, two digits after the decimal is enough. In fact, rounding (when presenting results, not when conducting the analyses) can often help your audience more easily understand your results.
- Avoid concluding that one result is “more significant” than another result because, for example, one p-value is 0.02 and the other is 0.0001. This is not a true statement. If you are interested in relative importance, you should consider effect sizes, but not p-values.
- “Almost” results. Avoid claiming that a result is “almost significant” or “nearly significant” when the p-value is, say, 0.055. No – your result is not significant. You can say that a result with a p-value = 0.055 is suggestive and that future research may want to follow up on this, but not significant is not significant, and you have to consider the role random chance played in obtaining that p-value.
- Non-significant results. Don’t spend time speculating why a result is not statistically significant. Due to the logic underlying hypothesis tests, you really have no way of knowing why a result is not statistically significant. Once you find that something is statistically non-significant, there is usually nothing else you can do, so move on and talk about something else.