Chi-square Tests
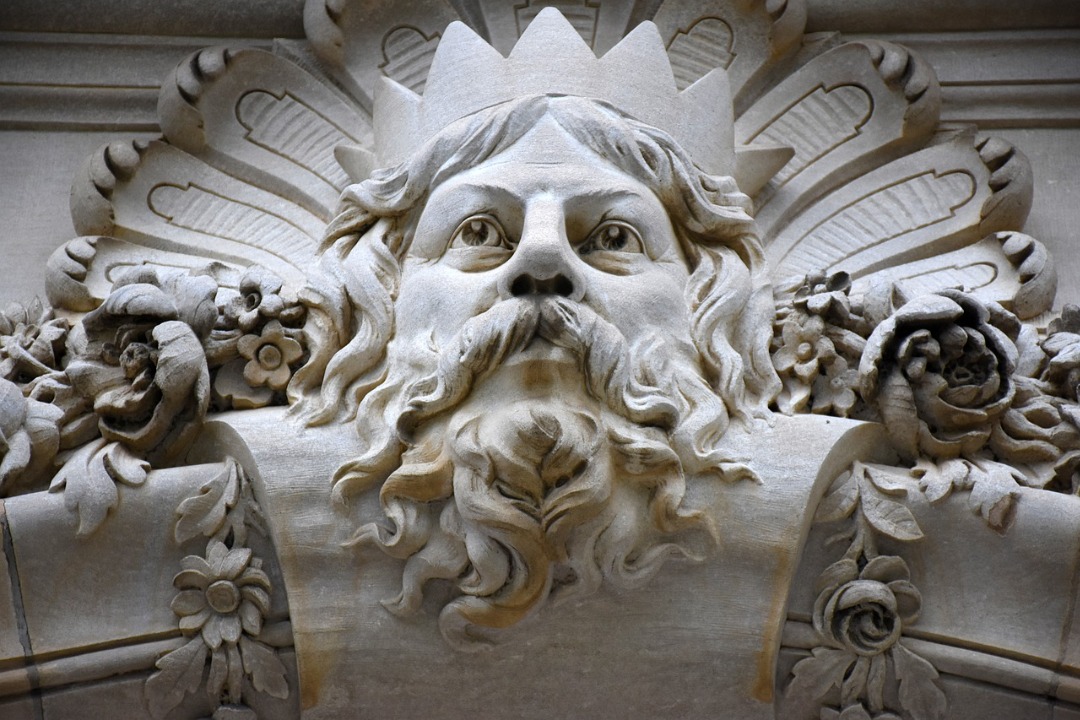
Chi-square tests look at the pattern of your data points and tell you if certain combinations of the categories occur more frequently than would be expected by chance, given the total number of times each category occurred.
This section explains the Chi-square Goodness-of-Fit test and the Chi-square Test for Independence.
- You want to compare your observations with expected values based on an assumed distribution (such as official statistics, published figures, etc).
- Your data is categorical data (see Types of Data for more information).
- You want to compare two – or more – sets of data to see if they are related.
- Your data is categorical data (see Types of Data for more information).
The Chi-square Goodness-of-Fit Test examines the discrepancy between the data we have observed and what we expect (based on an assumed distribution) and generates a measure of how significant that difference is.
The hypotheses for the test are:
H0: The observed distribution is the same as the expected distribution.
H1: The observed distribution is not the same as the expected distribution.
Two potential disadvantages of chi-square are:
- The chi-square test can only be used for data put into classes (bins). If you have non-binned data you’ll need to recategorize it before doing the test.
- It requires a sufficient sample size (n = 30) in order for the chi-square approximation to be valid.
An online calculator for this test can be found HERE.
Worked Example
You want to investigate whether your local community achieves the recycling rates boasted of by your country’s official statistics. The official statistics are given below in millions of tonnes per annum for 2022. You collect data from your local recycling centre for one week, in tonnes.
Does your observed data provide evidence of a difference in the recycling rates?
To address this question, we test the observed data’s goodness-of-fit to the official statistics. Before using the data we need to convert our data above (counts) into percentages (proportions), as below. Make sure your percentages total to 100%. For example, for the official statistics for glass you would calculate 91.7 ÷ 292.4 = 0.314 = 31.4% Using the online calculator HERE, we get a calculated p-value for this test = 0.82 which is greater than the critical propability or significance level of 0.05 (5%). So, we cannot reject H0. Therefore, we fail to reject the null hypothesis and conclude that there is evidence that your local community is recycling in line with the official statistics. |
The Chi-square test for independence looks for an association between two categorical variables within the same population. Unlike the goodness of fit test, the test for independence does not compare a single observed variable to a theoretical population but rather compares two variables within a sample set to one another.
The hypotheses for the test are:
H0: The variables in question are independent – they are not related.
H1: The variables in question are not independent — they are related.
An online calculator for this test can be found HERE.
PRACTICE - Test for Independence
Worked Example
You want to test whether the commitment to recycling is related to gender. You gather data by interviewing a random sample of 216 people and asking them about their recycling habits.
The data has been recorded and organised in the table below.
Construct a chi-square hypothesis test to determine if there is enough evidence to support your conjecture. Use a 5% level of significance.
The hypotheses for the test are: H0: Recycling is not related to gender. H1: Recycling is related to gender. NOTE: Gender is our two groups and the frequency of recycling is our categories. Using the online calculator HERE, we get a calculated p-value for this test of 0.039. Since this is less than the critical probability or significance level of 5% (P = 0.05), we reject H0. Therefore, we reject the null hypothesis and conclude that there is evidence that people’s recycling habits are related to their gender. |
Farms in your local are use three brands of fertiliser (A, B and C). You wish to test whether fertiliser brand has a significant impact on the crop yield. You collect data from 120 different farms, asking each farmer to classify their crop yields as high, medium or low. The data collected are shown in the table below.
We will use a Chi-Square Test for Independence to test whether there is a statistically significant difference bewteen the three brands of fertiliser.
What will our null and alternative hypotheses be for the test?
The Chi-square test for independence looks for an association between two categorical variables within the same population. So our null hypothesis is that the two variables (brand of fertiliser and crop yield) are indepedent.
We will use the online calculator HERE.
Which of the following statements are correct?
In this case, the brand of fertiliser will be the “group” and the yield will be the “category”.
Once the test is run, what can we conclude?
Since the p-value (0.425) is greater than the critical probability or significance level (P/α=0.05) we must fail to reject the null hypothesis.