ANOVA
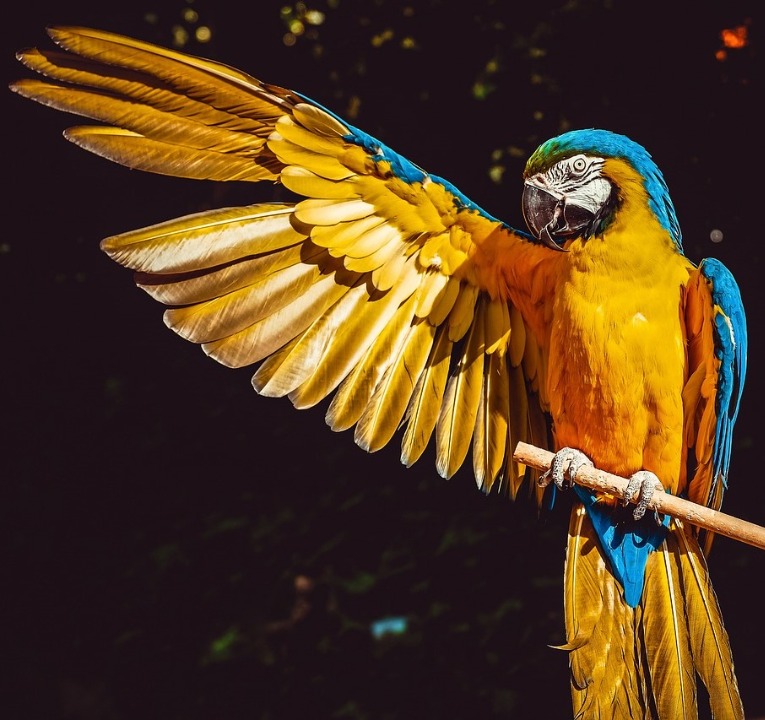
A one-way ANOVA (“analysis of variance”) compares the means of three or more independent groups to determine if there is a statistically significant difference between the corresponding population means. This section explains how to carry out a one-way ANOVA test and a two-way test.
If your ANOVA reveals significant results, then you should run a Tukey’s Honest Significant Difference Test to find out which specific groups’s mean is different. The test compares all possible pairs of means.
- Your data has been collected from three or more independent groups OR the same group tested under three or more different conditions.
NOTE: Each group does not need to be equal in size. - You want to test whether there is a statistically significant difference between the three or more populations from which these groups were drawn.
- Your data is assumed to be sampled from a normally distributed population.
NOTES:
The Kruskal-Wallis test compares the medians of three or more independent groups while a one-way ANOVA compares the means of three or more independent groups.
You can use a Shapiro-Wilk Test OR Kolmogorov-Smirnov Test to check for the assumption of “normality”.
A one-way ANOVA (“analysis of variance”) compares the means of three or more independent groups to determine if there is a statistically significant difference between the corresponding population means.
There's no minimum number of data points required to perform an ANOVA, but very small datasets make it is less likely that you will determine a difference in the means.
The following assumptions should be met:
- Normality – Each sample was drawn from a normally distributed population.
- Equal Variances – The variances of the populations that the samples come from are equal. You can use Bartlett’s Test to verify this assumption.
If these assumptions cannot be met you could do a Kruskal-Wallis test which compares the medians of three or more independent groups.
There are two sorts of ANOVA tests:
A one-way ANOVA test for independent measures – use this when your data is from independent groups. This means that different people are in each group.
A one-way ANOVA test for repeated measures – use this when your data is from the same set of individuals tested under three or more conditions.
A one-way ANOVA uses the following hypotheses:
H0 (null hypothesis): All the population means are equal (μ1 = μ2 = μ3 = … = μk).
H1 (alternative hypothesis): At least one population mean is different from the rest.
An online calculator can be found HERE for independent groups and HERE for repeated measures.
Two-way ANOVA is done when you want to know how two factors affect a response variable and whether or not there is an interaction effect between the two factors on the response variable.
For example, suppose a botanist wants to explore how sunlight exposure and watering frequency affect plant growth. She plants 40 seeds and lets them grow for two months under different conditions for sunlight exposure and watering frequency. After two months, she records the height of each plant. In this case, we have the following variables:
- Response variable: plant growth
- Factors: sunlight exposure, watering frequency
An online calculator can be found HERE.
Worked Example
A researcher wants to know whether different advertisements impact people’s views on recycling. She recruits 30 individuals and randomly splits them up into three groups to view either no advertising, Advertisement 1 or Advertisement 2.
After viewing, each individual completes a short survey asking questions about their views on recycling. The survey uses a 5-point Likert scale from “strongly disagree” (1) to “strongly agree” (5). Questions are worded such that “strongly agree” always indicates a positive attitude towards recycling.
Once all the surveys are completed each person’s total score is calculated, with a possible maximum of 25. You then have the following data:
No advertisement: 23, 12, 16, 9, 14, 8, 14, 5, 18, 13
Advertisement 1: 13, 22, 21, 19, 24, 6, 17, 15, 20, 19
Advertisement 2: 23, 22, 12, 9, 27, 16, 24, 5, 12, 9
Using an ANOVA, determine whether there is a statistical difference between the three groups.
The three groups are independent as different people were in each group, so we use a one-way ANOVA test for independent measures. The hypotheses are: The null hypothesis (H0): All the population means are equal. Using an online calculator we get a p-value of 0.28. Since this is more than our level of significance (Significance Level = 5% or p = 0.05) we cannot reject our H0 and must conclude that the means of the three groups are not statistically different, that is, these adverts do not seem to impact people’s views on recycling. |
After you have run a one-way ANOVA and found significant results, then you should run Tukey’s HSD Test to find out which specific groups’s mean is different. The test compares all possible pairs of means rather than comparing pairs of values.
The online calculator HERE will perform the Tukey's HSD Test for you after a one-way ANOVA.
You want to compare four different fertilisers and their effect on crop yields. You find five farmers who are willing to test each brand of fertiliser in separate fields and measure their crop yields (kg/ha).
You then have the following data:
Brand A: 8750, 9125, 7980, 7950, 8150
Brand B: 9225, 8765, 8945, 7490, 8450
Brand C: 7950, 8750, 8960, 8940, 8760
Brand D: 7890, 9870, 9560, 8560, 8760
Is the assumption of independence reasonable in this case?
It is reasonable to assume the data is independent because the farmers are testing the different brands in different fields. This means we can do a one-way ANOVA test for independent measures.
What would be the null and alternative hypotheses for this situation?
The null hypothesis (H0) is that all the population means are the same.
The alternative hypothesis (H1) is that at least one population mean is different from the rest. Some can be the same - but at least one is different.
Using the online calculator HERE, enter your data using a significance level (α) of 5% (p = 0.05).
"Treatment 1" will correspond to what in the data above?
In this example we have four "treatments" which correspond to the four brands we are comparing.
Which of the following are valid conclusions we can draw after running the test? Tick all that apply.
You now re-do your research and collect the following data:
Brand A: 18750, 19125, 17980, 17950, 18150
Brand B: 9225, 8765, 8945, 7490, 8450
Brand C: 17950, 18750, 18960, 18940, 18760
Brand D: 7890, 9870, 9560, 8560, 8760
Using the online calculator HERE, enter your data using a significance level (α) of 5% (p = 0.05).
Which of the following are valid conclusions we can draw after running the test? Tick all that apply.
Now look at the Tukey Test results.
Which of the following are valid conclusions we can draw? Tick all that apply.
In the online calculator, a blue value for Q in the Tukey results indicates a significant result.
In this example, T1 represents Brand A, T2 represents Brand B, etc (provided that is how you entered the data!). So we see that the pairwise comparison of T1:T2 (Brand A to Brand B) gives a significant result as it is blue.