Statistics - the p-value
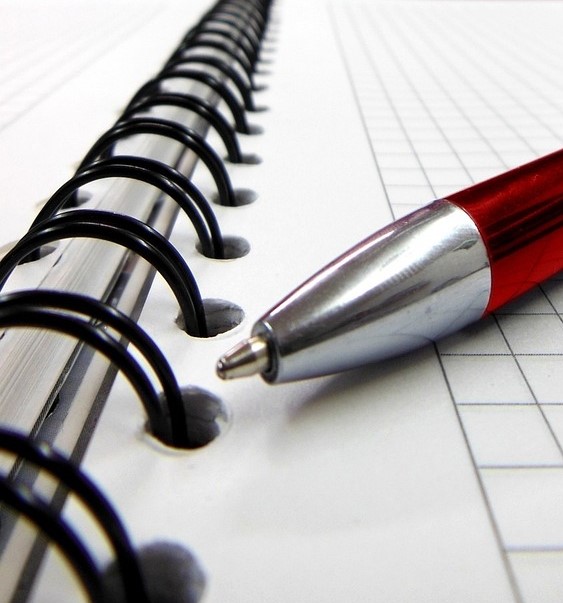
A p-value indicates how believable the null hypothesis is, given the sample data. Specifically, assuming the null hypothesis is true, the p-value tells us the probability of obtaining an effect at least as large as the one we actually observed in the sample data.
Your calculated p-value is compared to the critical probability (P) or significance level of your statistical test, which is typically set to 5% (p = 0.05). This gives a confidence level of 95% that if the research was repeated over and over again, the results obtained would be the same.
A p-value indicates how believable the null hypothesis is, given the sample data. Specifically, assuming the null hypothesis is true, the p-value tells us the probability of obtaining an effect at least as large as the one we actually observed in the sample data.
Here are a few other versions of its definition.
- The p-value is a number, calculated from a statistical test, that describes how likely you are to have found a particular set of observations, if the null hypothesis is true.
- The p-value is the probability, assuming the null hypothesis is true, that the result we have seen is solely due to random error (or chance).
When we compare two sets of data we use statistical tests to tell us the probability that the two sets are basically the same. This probability varies from 0 (not at all) to 1 (certain).
- The higher the probability, the more likely it is that the two sets are the same, and that any differences are just due to random chance.
- The lower the probability, the more likely it is that that the two sets are significantly different, and that any differences are real.
In ESS the critical probability (P) or significance level (α) is usually taken as 0.05 (or 5%).
This may seem very low, but it reflects the fact that ESS experiments are expected to produce quite varied results.
The confidence level of a statistical test is the probability that if the research/test/survey were repeated over and over again, the results obtained would be the same. It is also the probability that we make the correct decision about whether to reject or not reject the null hypothesis. We usually set this at 95%.
NOTE that the two values are related. The confidence level = 1 - significance level.
The smaller the p-value, the more likely you are to reject the null hypothesis, which is usually what we are hoping to do.
But how small is small?
If the p-value of your statistical test is lower than the critical probability (P) or significance level (α), it means your results are statistically significant and consistent with the alternative hypothesis (you reject the null hypothesis). You can reasonably assume that the two (or more) data sets you are comparing are different. If your p-value is higher than the critical probability (P) or significance level (α), then your results are considered statistically non-significant, and you fail to reject the null hypothesis. You can reasonably assume that the two (or more) data sets you are comparing are the same. | ![]() |
It is possible to make errors in your conclusions:
Type I error: You may incorrectly conclude that the null hypothesis is false when in fact it is true. This is what happens when you test positive for covid, but you don’t actually have it. This is called a Type I error. The risk of committing a Type I error is the significance level (α) you set at the beginning of your study. It is usually set at 0.05 or 5%.
Type II error: You may incorrectly conclude that the null hypothesis is true when in fact it is false. This is what happens when you test negative for covid, but you really do have it. This is called a Type II error. The risk of committing a Type II error can be minimised by increasing the sample size of your data.
When your results are statistically significant...
There is a temptation to consider this means that your results are ‘important’ and ‘meaningful’. But are they?
Consider an experiment that finds that the difference in mean height/length between the two groups to be 1cm and we find this difference is statistically significant (p=0.03). This means the average height/length in group 1 is different to the average in group 2 and this little discrepancy is probably not due to chance (based on the p-value). Even though this result is statistically significant, is it really important or meaningful?
The answer depends on the context and needs consideration. If we are comparing the heights of trees (with average heights of around 5m), a difference of 1cm in mean height between the two groups is probably not important... even though it is statistically significant. However, if we are comparing the length of worms (with average length of around 5cm) a 1cm difference would be a much more meaningful difference.
For this reason, it is crucial not to confuse statistical significance with importance.
When your results are not statistically significant...
Conversely, it is important to keep in mind that if an experiment fails to prove a statistically significant difference, there are two possible reasons for this:
- There actually is no difference.
- OR there is a difference, but the experiment didn’t detect it (a Type II error).
The first should not be considered a failure. Science is advanced as much by showing something didn’t work as expected, as by showing it did. The second is minimised through good experimental design.
When doing statistical test in ESS we usually set the following values...
Confidence level (%) =
Critical probability or significance level (%) =
We typically set the confidence level to 95%. This implies a critical probability or significance level of 5% since: Confidence level = 1 - critical probability
The confidence level is the probability that... (Tick all that apply)
In ESS we typically set this at 95% to reflect the fact that ESS experiments are expected to produce quite varied results.
When doing statistical tests, the calculated p-value is compared to...
We compare the calculated p-value to the critical probability or significance level which is usually 5% (0.05).