Statistical Tests
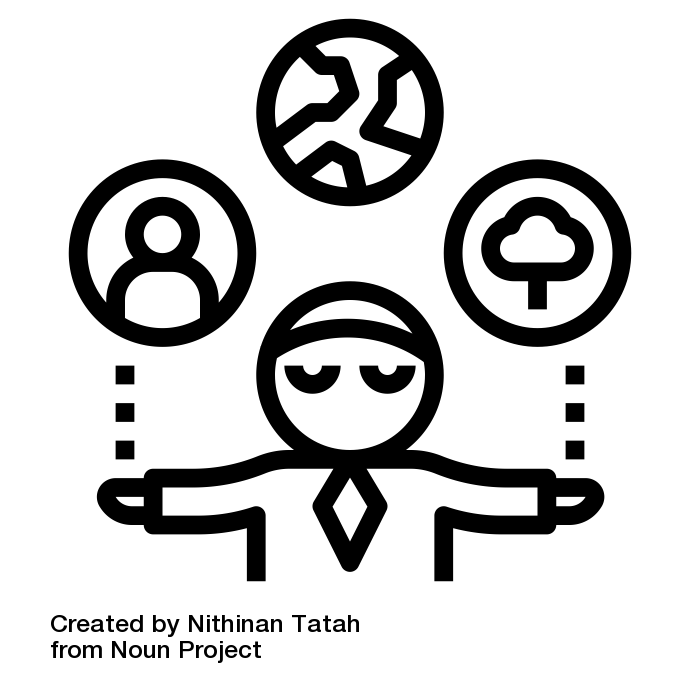
In this section we have a wide range of statistical tests that can be used to test your hypotheses. There is responsibility in collecting and presenting data and we need to be able to say if our findings are statistically significant or not.
While descriptive statistics are used to summarise data characteristics, inferential statistics go beyond this and are used to make predictions, prove or disprove hypotheses or find correlations/relationships based on data.
It can be very hard to know which statistical tests are the most relevant for your research question and your data. We provide a decision tree below.
Overview of statistical tests (with links) provides an overview of the different statistical tests, including our suggested online calculator for each test.
Developing Statistical Hypotheses
We always have a null hypothesis and an alternative hypothesis. We do statistical tests to decide which of the two hypotheses may be correct. This section explains how to develop your two hypotheses.
Statistics - the p-value
A p-value indicates how believable the null hypothesis is, given the sample data. Specifically, assuming the null hypothesis is true, the p-value tells us the probability of obtaining an effect at least...
ANOVA
A one-way ANOVA (“analysis of variance”) compares the means of three or more independent groups to determine if there is a statistically significant difference between the corresponding population...
Bartlett's Test
Many statistical tests (like a one-way ANOVA) assume that variances are equal across samples. Bartlett’s test can be used to verify that assumption.
Chi-square Tests
Chi-square tests look at the pattern of your data points and tell you if certain combinations of the categories occur more frequently than would be expected by chance, given the total number of times...
Kolmogorov-Smirnov Test
Some statistical tests ask you to assume that your data follows a normal distribution. The Kolmogorov-Smirnov Goodness of Fit Test compares your data to data from a normal distribution with the same mean...
Kruskal-Wallis Test
A Kruskal-Wallis test is used to determine whether there is a statistically significant difference between the medians of three or more independent groups. This test does not assume normality in the data...
Mann Whitney U-Test
A Mann-Whitney U-Test is used to compare the differences between two independent samples when the sample distributions are not normally distributed and the sample sizes are small (n < 30).
Pearson Correlation Coefficient
The Pearson correlation coefficient (also known as the “product-moment correlation coefficient”) is a measure of the linear association between two variables X and Y. It has a value between -1 and...
Regression
Regression is the statistical approach to find the relationship between variables. It allows you to estimate the value of a dependent variable (Y) from a given independent variable (X).
Shapiro-Wilk Test
Some statistical tests ask you to assume that your data follows a normal distribution. The Shapiro-Wilk Test compares your data to data from a normal distribution with the same mean and standard deviation...
Spearman Rank Correlation
Spearman’s rank correlation is a measure of the association between two variables. It has a value between -1 and 1. This calculation does not require your data to be normally distributed.
t-Test
The t-Test tells you how significant the differences between group means are. It lets you know if those differences in means could have happened by chance. The t-Test is used when data sets follow a normal...
Wilcoxon Rank Test
The Wilcoxon Rank Test is used to test whether there is a significant difference between two population means when your data cannot be assumed to be normally distributed.