t-Test
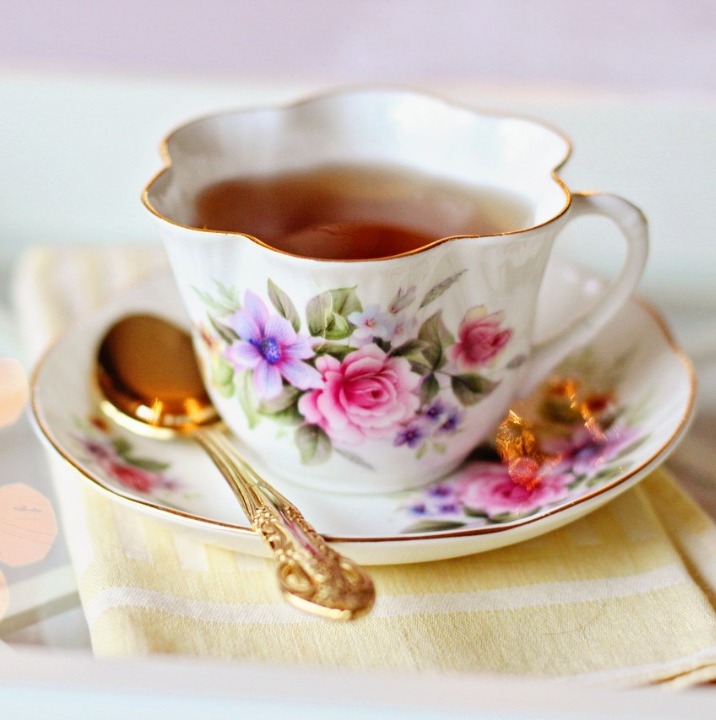
The t-Test tells you how significant the differences between group means are. It lets you know if those differences in means could have happened by chance. The t-Test is used when data sets follow a normal distribution.
- You want to compare two sets of data by comparing their means.
- Your data can be assumed to be normally distributed.
- You do not know the variances of the underlying populations.
- Your data sample is small (less than 30, but more than 5).
NOTES:
(a) You can use a Shapiro-Wilk (for data sets with up to 2,000 values) OR Kolmogorov-Smirnov Test (for large data sets with over 2,000 values) to check for the assumption of “normality”.
(b) If your data cannot be assumed to be normally distributed, you should use a Mann Whitney U-test (independent data) OR a Wilcoxon Rank Test (repeated sample groups).
There are three types of t-test:
- An Independent Sample t-Test or Unpaired t-Test, which is used to compare the means for two groups.
- A Paired t-Test, which is used to compare the means from the same group but at different times, such as six months apart.
- A One Sample t-Test, which is used to test a mean of a group against the known mean.
Before using the t-Test you will need to calculate the means and standard deviations of your data groups. See HERE.
The t-test statistics are based on the t-distribution, which is symmetrical and bell-shaped like the normal distribution, but has heavier “tails”. This means that any confidence intervals derived from it will be wider than from the normal distribution. Since we have small sample sizes, we’re less certain about the true population mean so it makes sense to use the t-distribution to produce wider confidence intervals that have a higher chance of containing the true population mean.
An online calculator for raw independent data can be found HERE. And for summary data (where you have calculated the means and standard deviations of your data HERE.
An online calculator for raw paired/repeated data can be found HERE.
Worked Example 1
A comparison of the temperature of sea water was made at two locations. Over a 2-month period, daily readings were taken (n = 60 readings in total at each location). Descriptive statistics were then calculated for each location:
Location A: mean (μ1) of 22.4° with a standard deviation (s1) of 1.6°.
Location B: mean (μ2) of 24.3° with a standard deviation (s2) of 1.8°.
Does the average temperature at the two locations differ? Test at the 5% level of significance.
Here we are testing the following hypotheses: H0 : μA = μB (ie that the two locations have the same mean) H1 : μA ≠ μB (ie that the two locations have different means) This is an two-tailed independent sample t-test as we used two separate locations and we are testing for a difference between the means (either larger or smaller). Since we have the summary data, we can use the online calculator HERE. We find that t = 6.111 and p-value = 0 As the p-value for this test is zero, we have compelling evidence against H0. Therefore, we reject the null hypothesis and conclude that there is evidence that the sea water temperatures at the two locations is significantly different. |
Worked Example 2
You believe there is an increase in the number of younger people choosing to use trains for their vacations (rather than planes). To test your theory, you sampled students after the summer holidays and asked how far they had travelled on trains over the holiday period. You then compared the results to another survey that was done four years ago.
Results are shown below:
Old survey: n1 = 125, mean distance travelled on trains (μ1) = 760km, std dev (s1) = 165km.
New survey: n2 = 159, mean distance travelled on trains (μ2) = 880km, std dev (s2) = 235km.
What can you conclude? Use a 5% level of significance.
Here we are testing the following hypotheses: H0 : μold = μnew H1 : μold < μnew This is a one-tailed independent sample t-test with unequal variances. Since we have the summary data, we can use the online calculator HERE. The test shows that the means for two groups are significantly different from each other at 5% significance level. Therefore, there is sufficient evidence to conclude that students have changed their travel patterns. |
The growth of plant seedlings in two different types of soil was measured. We want to know if growth was better in Soil Type 2. Growth (in cm) over a year was measured for 10 plants in each type of soil and is shown below. Test at the 5% level of significance.
Soil Type 1: 3.2, 4.5, 3.8, 4.1, 3.6, 3.1, 7.2, 4.9, 6.4, 7.8
Soil Type 2: 4.5, 6.3, 5.7, 6.0, 7.2, 5.8, 9.2, 8.4, 6.7, 5.3
As we have raw data we will use the online calculator HERE.
Match up the following for the test.
Treatment 1 | 0.01 |
Treatment 2 | Two-tailed |
Significance level | Soil Type 1 |
One-tailed or two-tailed hypothesis? | Soil Type 2 |
One-tailed | |
0.05 |
We want to test whether Soil Type 2 is better than Soil Type 1 which is a one-tailed test.
The test produces a LOT of information... but scroll down and you should see:
"The p-value is .029864. The result is significant at p < .05."
What can we conclude from this? Tick all that apply.
Our rule of thumb is ... "If p is low, H0 must go". This test has resulted in a p-value less our critical probability or significance level of 5% so we reject the null hypothesis.