Identifying bias in an AI world
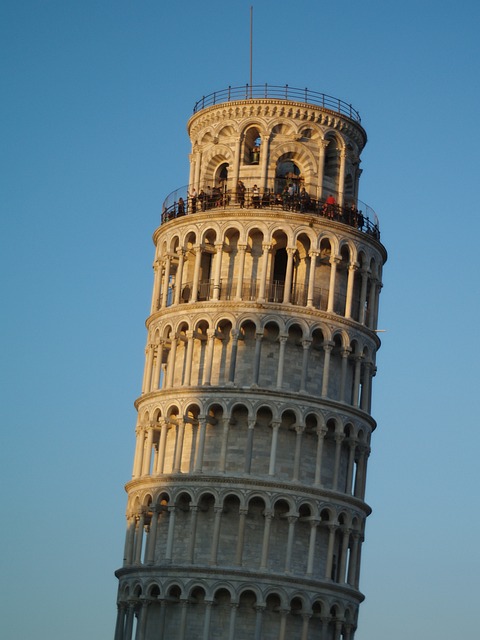
Never assume impartiality; always be aware
The identification of bias and the implications of such has gathered significance in the 2020s with the emergence of AI. We must understand the capacity for bias in algorithms and how it comes about as well as the presence of bias in AI detection software as well. The secret seems to be awareness of the potential for bias and never to assume impartiality. Here we explore ways to identify bias, the ethics surrounding AI and case studies to take understanding further
The irony is not lost that Artificial Intelligence shares initials with Academic Integrity. Schools everywhere have adapted to the existence of this tool which, when used as a tool, can assist the learning process hugely. However, it can also be misused and abused too.
Bias can be difficult to spot
It's often not a hammer over the head so you cannot look out for that; it is often subtle and unintentional. However, there are a few key indicators to look for.
First of all, look for any type of generalization or sweeping claims that make broad statements about certain demographic groups or cultures. Secondly, look for any type of loaded terminology or words used to manipulate the reader into having a certain opinion. Finally, check if the author cites multiple sources to back up their claims, or if they just rely on a single source. Overall, the best way to spot for bias is to become familiar with the various biases that exist and to be an active reader. Question the source, read multiple perspectives of an argument and be aware of any rhetoric used to persuade or bias the reader.
Remember the Raven process when researching
Overview
Artificial Intelligence is a vast area of ethical debate which branch off into all sorts of dilemmas. Just one area is the role of bias in AI algorithms and the effect that this has had. It is interesting to consider the impact and influence of this as a way of developing expertise in criterion B of the Reflective Project.
The ethical implications of algorithms and Artificial Intelligence (AI) are of great importance, especially when it comes to AI bias. AI bias is when an algorithm is trained to interpret data in a certain way and produces results that are inaccurate, unfair, or discriminatory. This can lead to discriminatory outcomes, such as the exclusion of certain groups of people from products and services.
AI bias can also lead to a lack of accountability, as it is often difficult to trace the source of the bias. Additionally, data collection and training methods can lead to a lack of transparency, making it difficult to detect or correct bias in algorithms. To prevent AI bias, companies must ensure that the data used to create algorithms is valid, unbiased, and representative of the population. Companies must also have a clear understanding of their algorithms and the data used to create them. Finally, companies should also strive for transparency by providing access to their algorithms and data, and allowing for independent auditing of their algorithms.
By understanding and addressing the ethical issues of AI bias, companies can ensure that their algorithms are fair, accurate, and free from discrimination.
What is AI bias?
To put it more simply ...
'AI bias is an anomaly in the output of machine learning algorithms, due to the prejudiced assumptions made during the algorithm development process or prejudices in the training data'
Source: British Medical Journal 'Cascading effects of health inequality and discrimination manifest in the design and use of artificial intelligence (AI) systems'[1]
Case Studies to explore
Here are three well-known examples of ethical issues of bias in algorithms.
a) Explore the who, what, when and where
b) Then think about how this happened and why it has implications. Think about the 'so what?'
c) Then consider solutions that may or may not have been provided for this issue. What is next for this issue? Also think about future use of AI and the potential for bias?
1. COMPAS (Correctional Offender Management Profiling for Alternative Sanctions) algorithm
Teacher notes: A little more
One of the most famous cases of AI bias is the COMPAS (Correctional Offender Management Profiling for Alternative Sanctions) algorithm [1]. This algorithm has been found to be biased against African American defendants, who are more likely to be classified as high-risk than whites, even when the risk scores are similar.
The COMPAS algorithm has been heavily scrutinized for its ethical implications, particularly in the context of racial discrimination and mass incarceration. This algorithm is used in many U.S. states to predict the likelihood of a defendant's recidivism and inform sentencing decisions. However, studies have found that the COMPAS algorithm often produces different results for Black and White defendants with the same criminal histories, and that Black defendants are incorrectly classified as higher-risk more often than White defendants.
Given the ethical implications of this algorithm, many civil society organizations have called for its suspension or abolition. They argue that using a biased algorithm to make decisions about an individual's fate is fundamentally unjust and that alternative methods should be explored.
One solution to this problem would be to use a fairer algorithm that takes into account a variety of factors, such as criminal history, social context, and community resources, to generate a comprehensive and unbiased risk assessment. This algorithm should also be regularly reviewed to ensure accuracy. Additionally, individuals should have access to the algorithm's results and have the option to challenge incorrect predictions.
2. US Healthcare system
Teacher notes: A little more
Bias in algorithms used to power healthcare decision-making in the United States is both a serious issue and a hidden one. While healthcare is meant to be provided on an equitable basis, algorithms used in decision-making can often propagate inequity and lead to preferential treatment for certain groups. There are multiple sources of bias that can lead to unequal outcomes, such as the language used in data sets and the data points used to build the algorithms. If the data set used to train the algorithm is not diverse enough, it is more likely to perpetuate existing biases in the population, leading to unequal outcomes.
Another source of bias can be the design of the algorithm itself. If the algorithm is designed to favor certain outcomes, it could lead to unequal treatment for minority groups. Furthermore, if the algorithm is designed to favour certain types of treatments, it could lead to cost savings for the healthcare provider but may not be in the best interest of the patient. Finally, bias can also be caused by the way the algorithm is used. For example, if there is a bias toward certain treatments, then those treatments may be used more frequently than the others, leading to unequal outcomes.
To combat bias in healthcare algorithms, many organizations have started to use transparency and accountability measures. This includes making the algorithm open source and providing detailed explanations for why certain decisions were made. Additionally, the use of external reviews and audits can help to identify any potential bias and ensure that the algorithm is working to promote equity in healthcare decision-making.
3. Amazon's hiring system
Teacher notes: A little more
'Amazon’s one of the largest tech giants in the world. And so, it’s no surprise that they’re heavy users of machine learning and artificial intelligence. In 2015, Amazon realized that their algorithm used for hiring employees was found to be biased against women. The reason for that was because the algorithm was based on the number of resumes submitted over the past ten years, and since most of the applicants were men, it was trained to favor men over women'
Footnotes
- ^ British Medical Journal, 2021, retrieved from https://www.bmj.com/content/372/bmj.n304